#134 WaveForm AI
Subscribe to get the latest
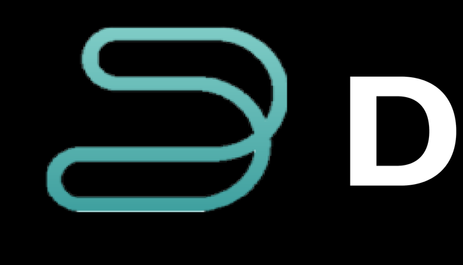
on Wed Apr 19 2023 17:00:00 GMT-0700 (Pacific Daylight Time)
with Darren W Pulsipher, Logan Selby,
In this episode Darren Pulsipher, welcomed Logan Selby, the co-founder and president of DataShapes, where they discuss a unique approach to Artificial Intelligence that is bucking the trend.
Keywords
#artificialintelligence #waveformai #datashapes #waveform #technology
Listen Here
Logan is a former intelligence officer in the DOD and has a passion for AI and robotics, which led him to be a reservist in the defense community. He transitioned to DataShapes, where they have a unique technology that solves critical needs in the defense community using AI. While AI has been around for a long time, the vast amount of data available for training models and the advancement of computing resources have led to the development of smarter systems like chatbots and large language models.
Current techniques are resource-hungry and very costly to train and build a general-purpose inference solution. For example, running large language models like ChatGPT can cost up to $3 million a day, but AI is evolving rapidly and receiving more attention than ever. One concern in using neural networks is the inability to audit and explain how the AI arrived at a result. There are social, political, and legal challenges to trusting decisions made by these networks, particularly in fields where human life is at stake. However, society will eventually overcome these challenges and fully embrace AI. DataShapes’ approach, which uses traditional machine learning techniques to solve pain points in data analysis, has full auditability and discovery in their trained models.
DataShapes has a unique approach to solving problems quickly and efficiently in resource-constrained environments. While traditional neural network training requires a lot of labeled data and can be brittle, DataShapes uses methodologies to learn in real-time or near-real-time. Their technology focuses on waveforms and signals and is auditable, making it ideal for use in austere environments where people are getting dirty and carrying a server stack is impossible. While neural networks are great at language models and image recognition, Logan’s company is hyper-focused on waveforms and signals. Their approach is different and highly effective.
Detecting different types of waveforms and relationships in the waveforms is at the center of this new technology. This approach detects patterns harder to spoof compared to traditional techniques used by the Department of Defense. This has the potential for applications of their technology in electronic warfare, including gathering intelligence and analytics. Additionally, the platform can detect, analyze, and gather intelligence, which can be exported to edge devices. Their self-learning anomaly detection feature, Infinite Loop, was also mentioned, which establishes a continuous baseline based on the parameters the end-user prescribes. The technology can be used in the automotive industry, healthcare, and entertainment industries, where it could be embedded in every sensor.
DataShapes has a product called GlobalEdge, an intelligent agent that sits on or behind sensors to conduct ETL operations for data being collected. The machine learning component of GlobalEdge filters the data to provide relevant insights and anomalies in real-time, reducing the amount of irrelevant data being pushed back to headquarters. The product can also be used for data compression on the edge to the data center. The software can scale down to as little as 47 K, making it suitable for a variety of applications, including virus detection using UV waves.
To find out more about DataShapes and their approach check out https://www.datashapes.com
Podcast Transcript
1
Hello, this is Darren
Pulsifpher, chief solution,architect of public sector at Intel.
And welcome to Embracing
Digital Transformation,where we investigate effective change,leveraging people processand technology.
On today's episode Way for me,
I was a special guest, Logan Selby'sco-founder and president of DataShapes.
Logan, welcome to the show.
Thanks, Darren. Appreciate.
I appreciate you.
And thanks for having me on.
Hey, Logan, we had dinner the other night.
We were thrown together at a dinner table.
We didn't know each other.
We sat next to each otherand we got to talkingand I went, this is super cool stuff,what you're doing.
But before we get into that, let's talka little bit about your background.
Where do you come from?
Logan Give me like a two or three minute.
What's where?
Who is Logan? Where do you come from?
Yeah, Yeah. No, absolutely.
So I spent most of my careerin the intelligence community in and DOD,mostly as an intelligence officer.
I spent time overseas.
I lived overseasfor an extended period of timein Germany and then didseveral different deployments.
I supported a bunch of differentorganizations throughout the intelligencecommunity during my time.
I'm still actively involvedon the defense side.
So currently athe lead for a time in roboticsfor organizationcalled the 75th Innovation Command.
We're a direct reporting unitto Army Futures Command,but we're reserve elements.
So I'm a reserve officer in the U.S.
Army, but I left full timegovernment service back in 2018 and spentsome time in the Fortune 500 environmentand worked for some startupsin the robotics communityand about 2000 in 2020.
I started advising for data shapesas a defense advisor because weat that point in timeknew that the technology, the data shapeshad was a perfect fitfor the defense community.
And then we got an injectionof capital in 2021,which allowed me to come on fulltime to run the company in 2022.
So I've been a data shapesince full time, since January of 22.
But on the academia side,you have a master's degree in data scienceand applied machine learning and a Ph.D.focused on autonomous systems.
So hence my sense, my attractionto robotics for the for the DOD.
But do do a lot of work stillfor the Army, like I said, as a reservist.
So I'm actively involved inwhat's going on in thethe autonomous systems and roboticscommunity throughout industry,academia and throughout the DOD.
Oh, that's awesome.
Now, whywhy move away from super cool robots?
So I've always data shapes.
Yeah,
I've always had an attraction for AI.
Like I said on Met,my master's program was really focusedon applied machine learning.
So I have a huge attraction toto that side of the business,which I wouldn't say is fully separatedfrom the robotics community.
But oh, no. No, it's it's tied. To it.
Definitely hardware versus softwareequation there.
But but no,data Shapes has a very unique technologythat solves a very critical needin the defense communityand throughout some other industriesthat I saw right away.
So it made sense for me to come aboard.
I felt like I could really push itand get it to the place it needed to be.
And we're we're thriving.
So we're we're finding our placein the world and in turning a lot of headsdoing so.
All right.
Before we get into ittoday, let's talk about AI in general.
When people hear about A.I.,they're hearing about chat, GPT,generative AI, large language models.
It's all the rage right now, right?
And we knowchatbots can conquer the world, right?
They've already proventhat they scared everyone.
There's a moratorium. Supposedly.
No ones do it. A moratorium.
Everyone knows that.
That's just their wayof slowing everyone else down.
What are your opinions on just the AI A.I.in general?
And then I want to talk a little bitabout a year guys's approach,which is very different.
Sure. Sure.
You know, so A.I.has been around for a long time,even deep learning modelsthat people like to throw around now.
You know, deep learning has been aroundfor for a long time as well.
I think now, you know,we're at a place in societywhere the amount of data that's availableto be pushed through some of these modelsfor training is is is extremely vast.
So that's why we're gettingsome of these very, very smart systemslike Chad GPT that can do a lot of thesedifferent things and kind of on demandand the computing resourceshave have evolved to a place to wherethey're readily more readily available,
I would say to, to allow peopleto do these things.
But it still requires a lot of resources.
It's an expensive thing to run.
You know, I think Chad GPT cost somewherebetween 3 to $5 million a dayjust just to run it now then that's.
Just to spit out what we already know.
Exactly. Exactly.
So there's there's a lot to it,but I'm happythat AI is getting getting the attentionthat it is.
I I'm definitely not on the teamthat we need to stop it.
I think it's
I think it's evolving at a rapid pace.
And I think we have to havean understandingof how it's going to be used and what it'sgoing to use for and who's using it.
But I definitely don't think we needto put a moratorium on it at this point.
So I have a question about that,because most of most of the airthat we hear about today and peoplein industry and outside of industryhave always heard of neural networks.
Right?
We're listening to oh,we need the program to operatelike our brain operatesusing neural networksand the whole concept behind it.
That's the big push in AI today.
Would you say that's true, Correct?
Correct. Yeah.
So neural networks,you know, if you're an unfamiliarto the audience,you know, neural networks are essentially,you know, layered parametric equationsthat are stacked on top of each otherto to perform a dutyutilizing mathematics.
The problem with neural networks isyou don't really knowhow or why a decision is made.
So an input goes in.
It is it is worked through the networkand then you get an outputand it's really hard to tellwhy that output is thereor how it got to that conclusion.
You may be able to point back to the datathat the model was trained on to saypotentially why this output waswas given based on this training datathat was shoved into the model.
But there's really no auditability there.
So the EXPLAINABILITYis kind of nonexistent.
Well, yeah.
And I remember there were some court casesaround this specific CLE, right.
How can we trust a convolutedneural network?
How can we trust any of theneural networks that are out there?
Because I have no proof of accuracy.
I have no way of determininghow it got to the answer that it got to.
Exactly.
Especially on the defense sidein other industries as well.
You know, where you have life, limb,eyesite involved, you know, trusting,trusting a decision something's madethat could result in a kinetic typeactivity is is onethat you have to be extra cautious on.
And so having something that's not ableto be audited is troublesome.
Do you think do you think as a societyis going to be able to overcome that?
Obviously,we already have overcome some of that, butdo you think we'll ever get to the pointwhere we fully trust a neural networkor that that technique of AI, becausethere's more than one technique of A.I.?
That's what we're going to talk about?
No, exactly. Exactly.
I think I think we will as a society,
I think eventually we'll just assumethat risk and say,you know, hey, it's providing a servicewhen it comes to,
I guess, more on the consumer side.
I don't know if we'll ever get to thatplace on the on the defense side unlesswe see some,you know, 99.99% resultsstatistically speaking,
You know, but it could get there.
I think it could get close.
But I think we're still a ways away.
No network.
That's the big buzz of the day.
We even have chips at Intel that doneuromorphic processing because, I mean,that's that's where every all the researchwell, all the big money is right now.
But you guys have a different approach.
We do.
I love this approach because it'sa simple approach to me.
It's it's not following the crowd.
I love people that kind of go againstagainst the grain because you spit outand you have this wonderful new technologythat that does wonderful things.
So explain a little bitabout why you guys decidedwhen you first startedlooking at your use cases, whyyou decided to go this different routeinstead of the traditional neural network?
Well, sure, sure.
So, you know,data shapes is mature in its technology.
So we've been aroundor I would say data shapes as IPhas been around for about a decade.
So our original engineering teamgot togetheralmost ten years ago now and developedthe technology that we have today.
And so that when they first lookedat some of the pain pointsthat were around at the time,they realized that they could be solvedwith traditional machine learning.
So looking at youryour data science one on one, your Knearest neighbor type algorithms, supportvector machines, things like thatthat you hear about and in,like I said, data science one on one.
But they took that technologyand they evolved it several, several,several layers ahead, I would say.
And then and that's kindof where our secret sauce liesas far as our patents go.
But they found thatthose simple approaches were able to,number one, solve a lot of problemsquickly,efficientlyand in many different environments.
So, you know,ten years ago, your resource constrainedenvironments were even more so.
Nowadays, there's a lot more resourcesthat are available, but you stillpeople still need solutionsthat are on the edge, that are ableto be used in austere environmentswhere there's no networksthat where people are getting dirty.
You can't carry,you know, you can't jump out of aback of a plane with a server stack.
And so you know, you're going to needsomething that's able to betrusted, used in these environments,and that's extremely efficient.
And, you know, we'll talk a little moreabout this, but, you know,our solution is also auditable,which is another big factor.
Like I mentioned,when it comes to these kinetic typeactivities, you canyou can automate the entire workflow.
So you know why it's making the decisionthat it's making.
Exactly.
You know,from the initial training instance,all the waythrough the workflow to the output,you understand why the decision was madeand when and who trained it,and so on and so forth.
So this is interesting because you usesome of the same terminology that we usein, in traditional neural networktraining.
I'm training the model, right?
I'm doing inference.
You're using the same terminologies,but the underlying technologyis fundamentally different.
Correct? Correct.
Yeah.
It's it's thesome of the methods we use that,you know, people in the machine learningand AI community will understand iswe use a lot of zeroto few shot methodologies.
So we're actually learning in real timeor near real time.
So whatever dataset we're looking at,the data is coming in.
We're either using somethingthat supervised or an individualis actually looking at the data coming inand training that model in real time.
I always draw a boxbecause I think about our UI.
So enduser would be drawing a box around an itemand then telling the systemto learn it in real time versusyour neural net approach where it takesa lot of labeled data that's collecting,you know, thousands of imagesor thousands of,you know, whatever type of datathat you're trying to learn.
It takes a lot of it to feed itinto the model so it then can learn.
And then at the end of the day,that model's brittlebecause it's only as goodas the training data that you sent it.
So then if it's somethingif your output is wrongor it's incorrect, you have to go backand retrain that model and retrain it.
Yeah, it's hard to actually onand train a neural network.
Exactly. Soand so we're doing it in real time.
So if something is wrong,we can counter train in real timeor teach you something elseor don't show me this.
So that would be an example of a countercounter train. So don't.
Wait. So.
So why doesn'teveryone just use this stuff?
Why is everyonefocusing on neural networks?
Well, Ithink, you know, the Aurora methodologyisn't a panacea, I would say.
You know, there's definitelythe neural net solutions outthere are great at things,
I would say, and you're hearingabout a lot of them today.
So we talked about languagemodels, large language models.
GGP or image.
You know, neural networks are really goodat those thingsthat that technology,that science has been around for years.
So it's been perfectedstill resource heavy.
They they've come up with waysto get it a little smaller.
How we're utilizing our technologies,we're focusing ona completely different segment that othersdon't really talk about, and that'swaveforms, waveforms and signals.
Okay, So, so that'swhy that's why you can really focusis because you're saying
I'm not going to do a general AI right?
I'm going to focus on a specific type ofinput that comes into ISE,which is waveforms,correct? Correct. Yeah.
So we are hyper focused on on waveforms.
And when I when I say waveforms,you know, a lot of people think,especially if you're talking tolike somebody with a physics background,they'll say, well, images a waveformas well.
But you know, we're talkingmetaphorically,you know, the the the actual visualrepresentation of a of a waveformthat happens in the environment.
So, you know, you've EEG,radio frequency, vibration.
Even even voice, right?
Sound, even buoys sound. Yeah. Acoustics.
So that's that's the realm we play in.
And that's wherewe've really focused this technology.
That's really cool.
This kind of reminds me ofyou guys are like a specialist.
So if I, I come from a family of doctors,so I would not go to mymy brother, who's an oral surgeon,a specialist to have my appendixtaken out, even though I know he canbecause he's done general surgery rounds.
But if but I wouldn'tgo to a general surgeon toto have oral surgery done,my jaw replaced or whatever like that.
So you guys havehave you guys have special lostyour eye to certain types of problemsand input that that that you're looking atwhich I think is wonderful.
And that's a perfect analogy.
You know, sothere's there are some neural netbased solutions out there that have thatthat try.
And I think they do a decent job ofof waveform analysis.
But essentially the way they doit is through image.
So they arethey are taking the image of the way.
They take an image and drop. Yeah, yeah.
And so they're comparing itto other waveforms.
So it's it's very general.
So they're generalizing itjust in that process, let aloneso you know, the waywe're doing it using our technologies,we're actually digesting the waveform.
So we're taking what we call metrology,which are measurements of the waveform.
We're attaching metadata to that waveformin real time, which allows us to not onlylearn, learn everything that's happeningin the waveform, it allows us to query it.
So then if we run our AI throughany historical database of waveforms,you're able to do correlationsin real time of anything you've collectedhistorically as well.
Okay.
So let let's sounds super cool.
I know you guys are using vectorprocessingand things like thatbecause Intel's got vectorvector processing technologythat you guys can take advantage of.
And we talked a little bit about this,but let's, let's not go do too geeky.
I'm going to lose half the audienceif we do that.
Let's insteadlook at what can I actually use waveform
I forsure use cases.
I mean I mean you said sound inand anyyou know, in anything that produces a wavethat are waveformbut what can I practically use it for.
Yeah.
So so two two industriesthat we're working in right nowthat are complete polar opposites,one being defense.
You know, I've I've mentioned thata couple of times.
So on the defense side wherewe're working, we're working in signalsintelligence, electronic warfare,different types of acoustic signatures,things for on the intelligence sideof the house in defense.
But then we're also working in theentertainment industry just completely.
All right, Let's talk about entertainment,because this is going to be more in it.
Well, it'll be more entertaining.
Yeah, exactly. Exactly.
So we're doing a lot of work in music,so we're partner with somesome labels and some other organizationsthroughout the music industryto look at copyrightand artist attribution concerns.
And so.
Interesting.
Yeah, because it's that's, you know, audiolike you mentioned is a formand people don't necessarilythink about it like thatbecause there's a lot of solutionsout there that that try to compareaudio audio tracks for,you know, sampling and copyrightand things like that.
But the way we break down the waveformallows us to take it to the next level.
So there's issues in in music todaywhere even with this stuff,the generative AI stuff that's out now,there was an article that came out todayabout it,but generative, generative aside,you have social media influencers todaythat are there.
They're taking artists original tracksand then they're transforming themin a waythat can't be recognizedby other software.
And so they're taking,you know, yeah, let's say ten.
Other softwarethat's just straight pattern match.
Yes. Yeah.
So they're taking like a Taylor Swiftsong, for example, and putting it on itand putting it on there, their content,but they're transforming it.
So there's nothing that's attributingthat track back to Taylor Swift.
So there's, you know, royaltiesand all these thingsthat are owed to these artistsevery time their songs are usedthat they're not gettingbecause it's not able to be understood.
But the way our technology worksand the way we break down the waveforms,we actually learn it in a waythat we can pick out transformationsof the songs, which is is currentlyfrom our understanding.
There's there's a couple of companiesthat are dabbling in itout there,but we've really, really honed it inand have extremely,extremely robust solution.
So that goes to detection.
I'm hearing it does.
I can
I can use your I can use your technologyto detect different types of waveformsand relationships in the waveforms press,which is pretty slick,
I have to admit, becauseif we go to the Department of Defense,one of the techniques that people useis they use modulation or frequencyshiftingto get rid of of a to to spoofor confuse a guy from pattern matching.
But you guys could look at a relationshipthat's in the waveform itself,which would be harder toharder to spoof which. Correct?
Correct. Yeah.
So that's that's oneyou know especially when it comesto, you know, jamming and spoofing.
There's you know, I would say, you know,we've been in the Middle East for
So the the we haven't really had anear-peer adversary that we've came acrossup until now that has atechnology on the offensive sideof electronic warfare.
So that's a new, you know, areafor us too, that we're really trying todabble into,really pull more intelligence out of it
From a defensive perspective and analyticsperspective of electronic warfare.
There's a lot of intelligence thereto be gathered that's not not really beenexploited to date because there hasn'tbeen a software like ourspulling that that intelligenceout of the way for waveformreciprocal. All right.
So detection is that your main thing isjust I shouldn't say just detection.
It's a big deal.
Can I do any transformationfrom from these waveforms as wellthat you guys are detecting?
I mean, whatwhat other things can I do with it?
So so we areyou know, we have a pretty robust platformthat does the detectionbecause our software,our technology is so lightweight,we're actually ableto embed it on different things.
And so we have, you know,just for our product profile,you know, we have a softwarethat does the analytics,it does the detection, it does thereally the intelligence gathering.
It allows you to do correlation.
Then you can also create applicationsthat then can be exported to edge devices,and that software can be the mothershipsoftware,like we call it, the actual productwhere you're doing that can be doneon, you know, a ruggedized tablet.
It can be done, you know, on a laptop.
And then you can create these executablesthat can go downto the microprocessor level.
So so that that's coolbecause I can really pushapplications out to the edge,completely disconnected.
Correct.
And still get all of all of thatinformation, including self learning.
Right. Exactly.
So that's that's,that's one of our other productsthat that is that we call infinite loop.
So it is a a self learninganomaly detection, still detection,but it's essentially,you know, deploy and let it go.
So it's a self-learning applicationwhere it will establish a baselinecontinuously.
So based on the parametersthat an end user would prescribe.
So if you want to deploy it and have it,you know, listenor monitor or whatever,you're going to assign that duty to be.
It will continuously and self learnthat environment that it's deployed. Andthat's pretty.
Is there any way that these edge nodescan share their modelswith other edge nodes that are maybelisting in a different place?
Oh, absolutely.
Is there any way to correlatethose models together?
Because my brain is like going,
I could deploy this easilyinto a car that my teenagers are driving.
Exactly. Yes.
Because it's a way for the way they driveis absolutely a way for.
No, Absolutely.
It's fast. And slow. There's you know,there's everything. Right.
And I think, you know, speaking of,you know, vehicles, we've donesome use cases in the past and some POCswith the automotive industry,and that's been one of the use cases.
You know, the way the way that we'recollecting our data, you know, we canand way that we can be embedded,we can be embedded on every sensor.
So today, you know, the the averagethe average sensor count on a vehiclecoming off the assembly linetoday is like 80.
You know, that's that's average.
You know, Tesla probably being at theat the top of the range.
But you know, average is around 80.
And think of all the datathat's being collected constantly.
One of the issues, though,and another issue that we sawwith our technology is the the vastamount of data that's being collected.
There's not really a pipe big enough topush that data back or it will come off.
Because 5G is going to solve all that.
It would be cost effective.
No, you're right. Soso this is something that this issomething I've been touting as well.
I want to push analytics out to the edgeso I can still getall the valuable informationwithout moving all the data.
Exactly. Exactly.
So that's and that's one of ourother products we have called Global Edge.
And Global Edgeis essentially an intelligent agentthat sits on that sensoror just behind the sensorand it conducts, you know, youryour normal ETL operations.
So extract, transform and load of the datathat's being pulled at thatat the collection point.
But then our machine learningis on the back end of that, which actuallyreduces the data even moreand then filters it for the insightsthat the end user wants,which will allow you to pushthat real time intelligence back,whether it's on a vehicle, it's on a,you know,some type of defense collection platformor it's on, you know, a piece of machineryin a factory.
So you're you're actually getting the datathat you wantand kind of weeding through the noise.
So you're not constantly pushing streamdata back.
That's irrelevant.
Well, and so I have a question around thatbecause some people would say,but there might be something specialin that noise.
So we're able towe're able to capture that as well.
But you're able to captureall the anomalies in the noise, right?
Correct. Correct. Yeah.
We're able to capture any anomalies,any insights.
But then we can capture thatthat that big picture data too.
So it doesn't go away.
We can retain retainthe collection, the normal collection.
It just won't beobviously won't be pushed back to theto the headquarters in real timelike the insights would, or any anomaliesthat would pop up in the noise.
So thisalso helps with data compressionon the edge to to the data center.
I can have I can have what do we call it?
I've got a project
I'm working on now, has reinforcedcollaborative learning, reinforcecollaborative learningbecause I've got all these edge nodesthat arethat are out theredoing their own learning. Right.
But I want them to share. Exactly.
Yeah.
So we've we've ranthrough a couple of different exerciseswhere, you know, the way that the datais being pulled back with whateverwhatever data frameworkthat you want to ingest thisor digest this into because it doesn'thave to be our software, you know,we can plug it into whateverdata framework that you want, but we'vesince we have that self-learning,there are ways where you canyou can kind of cross-pollinate or sharethe learned data across your portfolio.
Across the portfolio, Yeah.
So super cool. Super cool.
You mentionedyou mentioned that it's small.
How small is small?
So the smallest to datethat we've scaled it down to is 47 K
Whoa.
Whoa, whoa, wait.
This will run on my 64 Commodore.
It will. It will.
So we havethat is pretty that's pretty cool.
So historically,you know, prior to getting into defensein entertainment,we actually worked a lot in health care.
And so we came up with some productsa few years agothat were looking at handheld PCR devices.
So, you know, mouth swab detection.
And we were looking to detect hepatitis Cand we were doing thaton a small little cartridge.
And so we're able to scale the softwaredown to around 4750 Kto make that detection.
So obviously the more complexyou would want your email ops or your Moperations to be, you would probably scalethat up a little bit.
But we can keep it factor.
You can keep it pretty.
So that's you just have another thingyou guys can do virus detection with this.
We can.
Yeah. So that's in that examplewe were detecting hepatitis
C just based on UV waves.
Being reflectors going to say Yeah. Yeah.
Yeah.
That's, that's pretty,that's pretty darn slick.
Now see, you guys have opened upthis big huge aperture for me because now
I'm thinking, what other crazy thingscan I do that that come in waveforms?
There's a lot of things that come inwaveformswe talked a little bitthe other night at dinner about imageand video processingand you said could do it. Butyeah, it's not optimized for it, right?
Not that'd be like going that'd be likegoing to my brother for an appendectomy.
He could do it,but he doesn't have all the right tools.
He hasn't done them in years.
So I want to go tosomeone that knows how to do that in.
Exactly. Yeah.
I mean, like I said,the science on those two areashave been around for a long timeand not not that it hasn'tbeen around on on waveform and that typeof environment too, but it's onethat we are obviously specializing inand that's whywe're trying to stay extremely focusedright now in defense and entertainment.
Are there are industries that we planto scale this out to down the road,one being I think we'll get back intohealth care eventually.
But energy is another onethat we're interested in down the roadbecause current is a waveform, you know?
Oh, absolutely.
The lights are currentat a very granular leveland we've tested thatand it works rather well.
So that's onethat we would like to get into eventually.
You know,
I have a feeling the defense worldmight drag us in that in that directionanyway.
But that's that's onewe're we're holding off now.
But we've been asked lately I've beengetting a lot of questions about verydifferent types ofwave forms that we don'tnecessarily experience on earth.
So a lot of like space, spacewave gravitational waves,you know, electromagnetic type wavesthat are being emitted in space.
So that's another another area that we'rebeing approached about, too, which is.
Maybe maybe we'll find Ceti.
Maybe. Yeah. Now that's maybe.
Yeah, that's one. There you go.
We would love that.
We would love to chat with.
I know mymy co-founder and I are very interestedin that area, so I think it would be funjust to have the conversation.
No, no, this,this is really, really cool stuff.
Logan
The conversationwe had a dinner just carriedon, on the podcast was just wonderful.
I appreciate you coming on the show.
You have anything else?
Where can people find outmore about data, shapesand and find out moreabout what you guys are doing?
Yeah, yeah.
So our website datashapes.com,you can look us up on there,you can requestinformation, request a demo.
We have a pretty active
LinkedIn profile as well,so you can check us out on LinkedIn.
I'm on LinkedIn,so feel free to reach out to me directly.
But we're we're tryingto build our presence.
Like I said, this is we really juststarted our go to market this year.
So we are just nowstarting our marketing campaign.
So a lot of peopledon't know about us yet.
So we're trying to spread the word andtrying to getout there and be a little more visible.
So, you know, well.
Most definitely you guys are someoneto watch in the future,even I would say watch rightnow. Don't wait.
Watch these guys.
I think I think you've got somethingunique here that is excitingand I most definitely am going to dosome more due diligence with you guys.
Well, I appreciate it, Darren,and thanks for having us on.
Thank you for listeningto Embracing Digital Transformation today.
If you enjoyed our podcast,give it five stars on your favoritepodcasting site or YouTube channel,you can find out more informationabout embracing digital transformationand embracingdigital.org
Until next time, go outand do something wonderful.